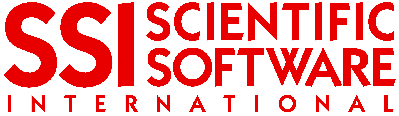


HLM 7 包括改進的介面、 HTML
輸出和新的統計功能,如交叉分類隨機效應線性模型三級資料,和四級資料線性和非線性模型。
階層線性模式 (Hierarchical
linear Model,簡稱
HLM),HLM所發展的階層模型(Hierarchical
Linear and Nonlinear Modeling)軟體,包含線性和非線性部分,HLM可以讀取大部份統計軟體的檔案如
SPSS, SAS, SYSTAT及STATA等等。HLM常用於社會科學和行為科學,因為它常有巢狀結構(Nested
Structure)的資料,因此需用次模型(Sub-Model)或階層模型(Hierarchical
Model),HLM就是設計來專門解決此類問題的,HLM提供的模型包括2-level
models、3-level
models、Hierarchical
Generalized Linear Models (HGLM)和Hierarchical
Multivariate Linear Models (HMLM)等。
HLM
7 includes an improved interface, HTML output, and new statistical
features such as cross-classified random effects for linear models
for three-level data, and linear and nonlinear models for four-level
data.
HLM
7 offers unprecedented flexibility in modeling multilevel and
longitudinal data. With the same full array of graphical procedures
and residual files along with the speed of computation, robustness
of convergence, and user-friendly interface of HLM 6, HLM 7
highlights include three new procedures that handle binary, count,
ordinal and multinomial (nominal) response variables as well as
continuous response variables for normal-theory hierarchical linear
models:
Four-level nested models:
Four-level nested models for cross-sectional data (for example,
models
for item response within students within classrooms within
schools).
Four-level models for longitudinal data (for example items
within time
points within persons within neighborhoods).
Four-way cross-classified and nested mixtures:
Repeated measures on students who are moving across teachers
within schools over time, or item responses nested within
immigrants
who are cross-classified by country of origin and country of
destination.
Repeated measures on persons who are simultaneously living in a
given
neighborhood and attending a given school.
Hierarchical models with dependent random effects:
Spatially dependent neighborhood effects.
Social network interactions.
HLM
7 also offers new flexibility in estimating hierarchical generalized
linear models through the use of Adaptive Gauss-Hermite Quadrature (AGH)
and high-order Laplace approximations to maximum likelihood. The AGH
approach has been shown to work very well when cluster sizes are
small and variance components are large. the high-order Laplace
approach requires somewhat larger cluster sizes but allows an
arbitrarily large number of random effects (important when cluster
sizes are large)
HLM 7 manual
A hard copy of the HLM 7 manual is not available.
PDF copies of the HLM 7 manual are available via the HLM 7
Manual option on the Help menu of the full, rental, trial, and
student editions of HLM 7 for Windows.
|